Start guides
Introduction
Openlayer is an evaluation tool that fits into your development and production pipelines to help you ship high-quality models with confidence.
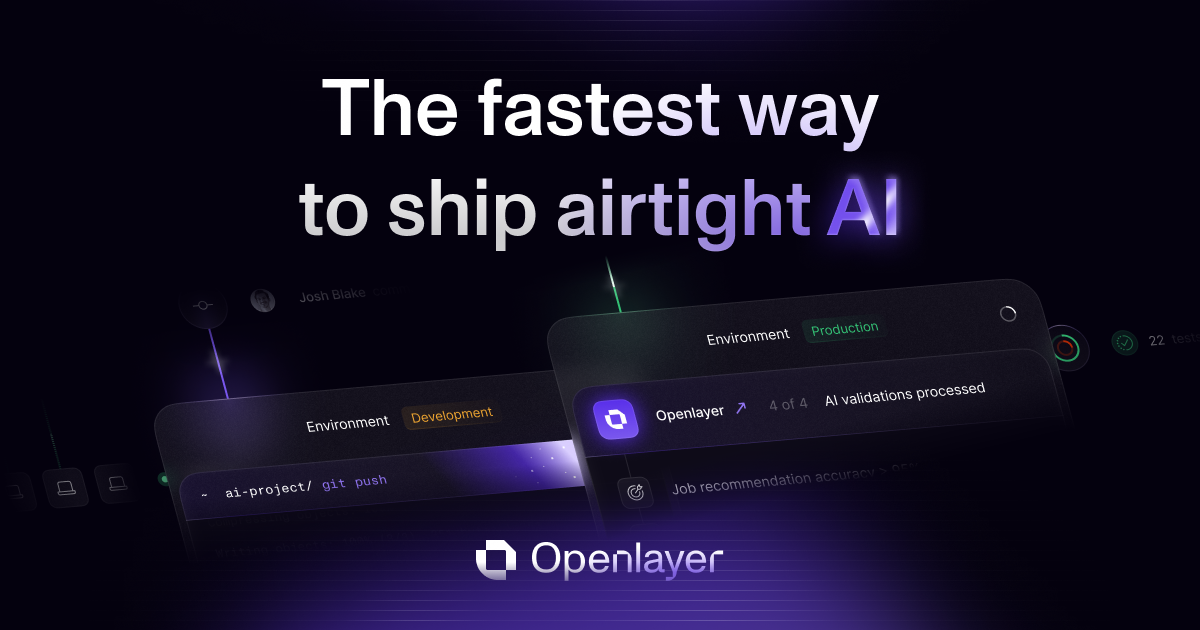
Start now
How this documentation is organized
Documentation
Full product documentation, to help you get set with Openlayer.
Guides
Straight-to-the-point guides that answer frequently asked questions.
Reference
Comprehensive description of Openlayer’s SDKs, CLI, and REST API.
Examples
Hands-on notebooks and sample apps with common AI patterns and tools.
Was this page helpful?